What Is Predictive Analytics?
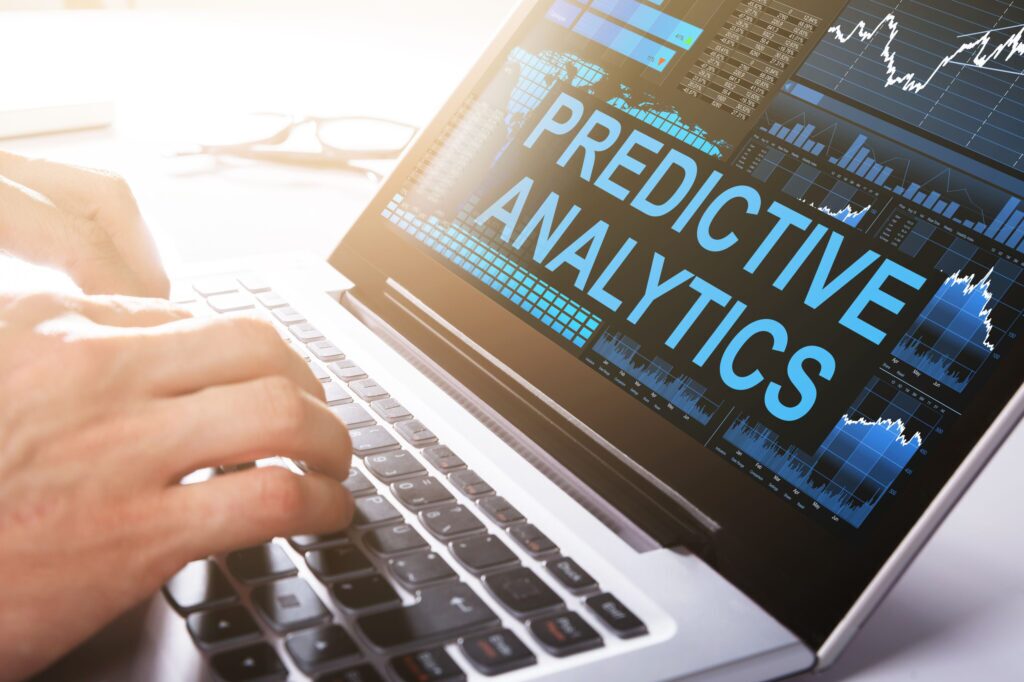
Predictive analytics is a mathematical method that makes use of data to determine patterns and trends for the purpose of predicting future outcomes. Whereas business intelligence focuses on describing current patterns and behaviors, predictive analytics focuses on possibilities and forecasts. Information gathered through predictive analytics is useful for anticipating market trends and finding opportunities.
How does predictive analytics work?
The practice of predictive analytics requires tools and techniques employed in various disciplines, including big data, predictive modeling, artificial intelligence, and machine learning.
Data is the most crucial element in predictive analytics. Using the entire history of captured interactions, behaviors, and transactions, a large body of data allows for more intelligent analyses of trends and patterns, and a more reliable model for forecasting future events.
Recent trends in technology and business have made predictive analytics possible and easily accessible to organizations, primarily through big data solutions. Domo predicts that by 2020, each person will be generating 1.7 MB of data every second. With customers constantly interacting with products in virtual environments and information being captured at every moment, data collection is now a standard process. Not all companies, however, have the capability to turn data into actionable insights–this is where predictive analytics comes in.
What are the benefits of predictive analytics?
An article by ZDNet reports that the top companies in the world now recognize the urgent need to invest in big data and AI solutions–not simply because of the benefits–but because of an increasingly competitive landscape, in which more data-driven companies stay ahead of the game. Here are some ways that businesses can become more agile and benefit from predictive analytics:
- Data-driven innovation and decision making – Product development efforts, implementation of changes, and market expansions would become more strategic endeavors rather than wasted effort because data-driven initiatives are more likely to succeed.
- Consumer insight – By analyzing customer behavior and preferences at a granular level, companies will have a more accurate understanding of their target market. This helps businesses anticipate needs and opportunities ahead of the competition.
- Operational efficiency – The benefit of predictive analytics is not limited to the analysis of externally acquired data. Many organizations use predictive analytics to measure and monitor employee and company performance. Data can also be collected to analyze productivity and gain insight into internal processes and personnel management.
What are predictive analytics’ examples?
- Marketing – Predictive analytics can drive growth for an organization. It can study consumer behavior, further segmentize its different markets, and analyze why certain campaigns work for certain groups over others. Then using that data, it can spot recurring purchasing or shopping behavior patterns from its customers and glean certain insights that can help the new marketing campaigns to be more attractive to their target markets. It can ‘predict’ which products or services will appeal to the customer and make the necessary recommendations. One clear example is the list of top picks of books that Amazon.com puts up on the home page of your Amazon membership page, and all this literature is based on the kinds of reading material that you had purchased from them previously.
- Healthcare – There are various applications of predictive analytics in the healthcare industry. Some examples would be the analysis of data to aid in diagnoses, predicting outcomes of certain procedures and medications, as well as calculating the likelihood of disease.
- Retail – A common application of predictive analytics can be found in online retail where businesses analyze user data on websites or mobile apps to determine interests and purchasing behavior. But this is not limited to online retail; physical stores also often use data mining techniques to understand buying patterns.
- Manufacturing – Quality assurance and maintenance in manufacturing require the analysis of equipment performance and the likelihood of defects. With the large scale of production, much of the analysis of processes need to be automated predictive analytics methods and software.
- IT Security and Compliance – Efforts to combat cyberthreats often involve predictive analytics to detect anomalies and unusual activity in a system. Using machine learning, cybersecurity solutions can anticipate possible attack points.